Which Technology Hides The Existence Of Data
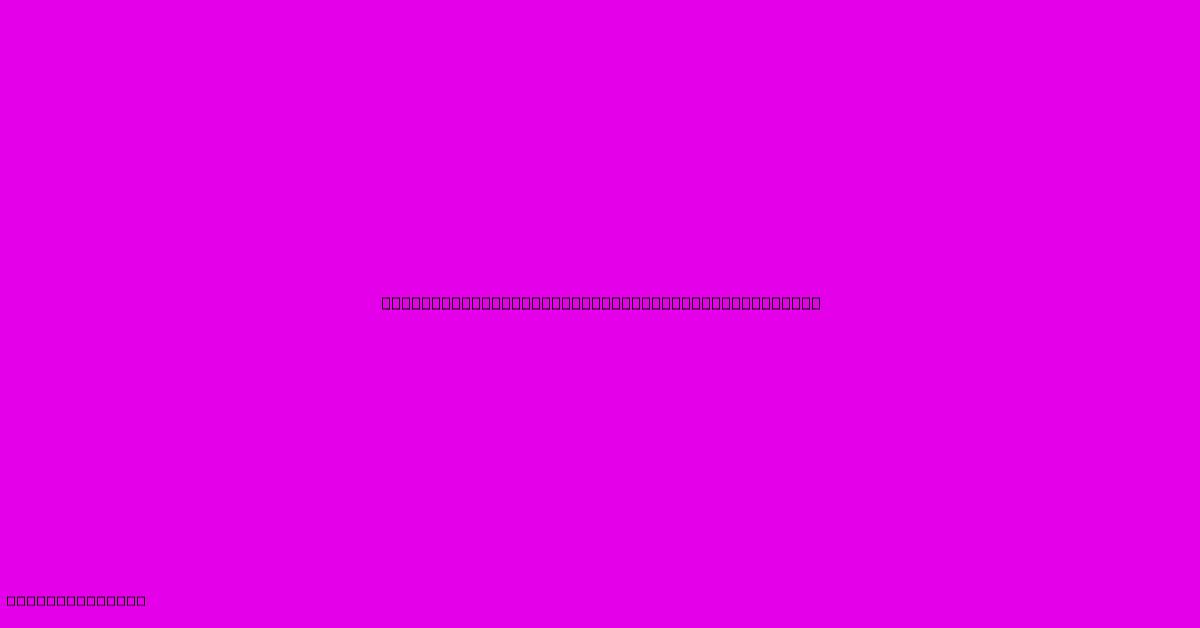
Discover more detailed and exciting information on our website. Click the link below to start your adventure: Visit Best Website mr.cleine.com. Don't miss out!
Table of Contents
The Stealthy World of Data Obscuration: Technologies Hiding Data in Plain Sight
The digital age is defined by data. But what if the very existence of data could be hidden? This isn't science fiction; several technologies are actively employed to obscure data, making it practically invisible to unauthorized access. Understanding these methods is crucial for both protecting sensitive information and navigating the complexities of data privacy in our increasingly interconnected world.
1. Steganography: Hiding Data Within Data
Steganography, a technique older than cryptography, focuses on concealing the very existence of a message. Unlike cryptography, which scrambles data to make it unreadable, steganography hides data within seemingly innocuous carriers like images, audio files, or even videos. This creates a "hidden channel" for secret communication.
-
How it works: Data is encoded into the least significant bits (LSBs) of the carrier's data stream. These slight alterations are practically invisible to the naked eye or standard analysis tools. For example, a seemingly normal image can secretly contain a hidden text message.
-
Strengths: Highly effective in avoiding detection; the data's presence is not even suspected.
-
Weaknesses: The carrier file must be large enough to hold the hidden data; sophisticated steganalysis techniques can detect anomalies.
2. Homomorphic Encryption: Computing on Encrypted Data
Homomorphic encryption allows computations to be performed on encrypted data without needing to decrypt it first. This means sensitive information can be processed and analyzed while remaining completely secure.
-
How it works: Special algorithms enable mathematical operations on ciphertext (encrypted data), yielding results that, when decrypted, are equivalent to the results of the same operations performed on the plaintext.
-
Strengths: Preserves data confidentiality even during processing; allows for cloud-based data analysis without compromising security.
-
Weaknesses: Computationally intensive, impacting performance; still a relatively nascent field with ongoing development.
3. Differential Privacy: Protecting Individual Data Points
Differential privacy adds carefully calibrated noise to data sets, making it impossible to identify individual data points while still preserving aggregate statistical properties.
-
How it works: Random noise is injected into the data during aggregation, making it difficult to discern individual contributions. The amount of noise is carefully calculated to balance privacy with data utility.
-
Strengths: Strong privacy guarantees; allows for the release of valuable statistical insights without revealing individual identities.
-
Weaknesses: Introduces a level of imprecision into the data; requires careful parameter tuning to balance privacy and accuracy.
4. Secure Multi-Party Computation (MPC): Collaborative Computation Without Data Sharing
MPC allows multiple parties to jointly compute a function over their private inputs without revealing anything beyond the output of the function.
-
How it works: Sophisticated cryptographic protocols enable collaborative computation without direct data exchange. Each party retains its private input.
-
Strengths: Extremely strong privacy guarantees; ideal for collaborative data analysis involving sensitive data.
-
Weaknesses: Complex to implement and computationally expensive.
Conclusion: A Balancing Act
The technologies described above represent a powerful toolkit for concealing data. They offer various trade-offs between security, performance, and data utility. The optimal choice depends heavily on the specific context and the sensitivity of the information being protected. As data privacy concerns continue to grow, the development and adoption of these technologies will undoubtedly play a crucial role in shaping the future of secure data handling. The challenge lies in striking a balance between the need for data security and the legitimate use of data for research, analysis, and innovation.
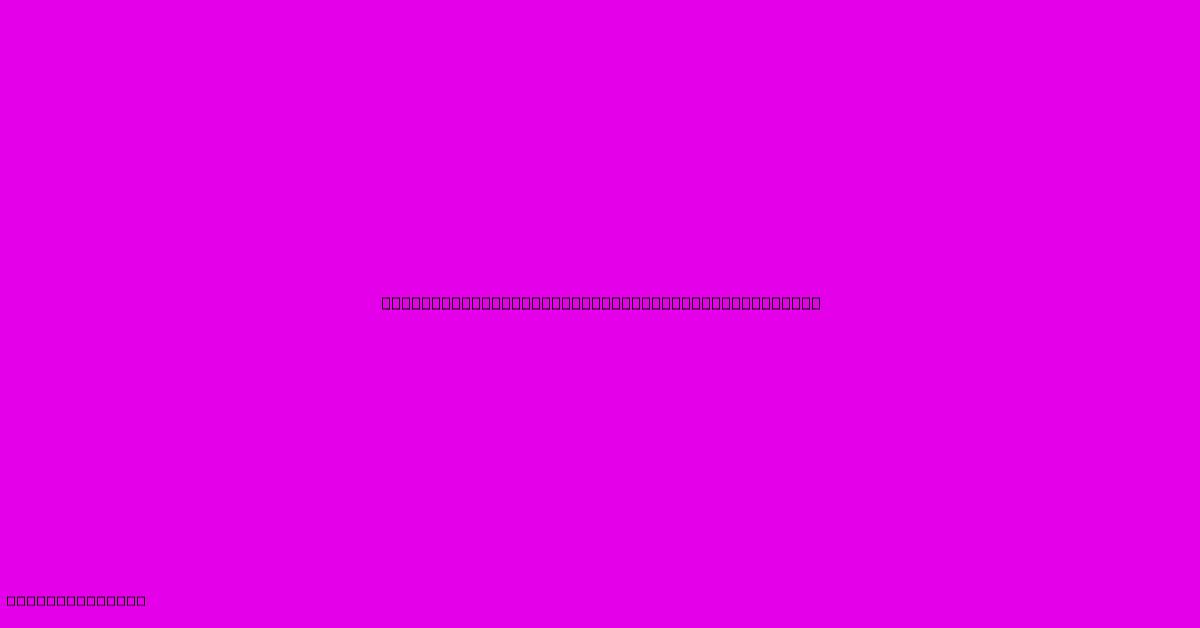
Thank you for visiting our website wich cover about Which Technology Hides The Existence Of Data. We hope the information provided has been useful to you. Feel free to contact us if you have any questions or need further assistance. See you next time and dont miss to bookmark.
Featured Posts
-
Tennessee Technology Center Alabama Avenue Memphis Tn
Dec 22, 2024
-
Barcelona Vs Atletico Hora Y Tv En Colombia
Dec 22, 2024
-
Mahomes Stroud Post Game Talk
Dec 22, 2024
-
Live Barcelona Vs Atletico Madrid La Liga
Dec 22, 2024
-
Aston Villa Man City Diepe Crisis
Dec 22, 2024