The Information Phase Of Technology Primarily Requires:
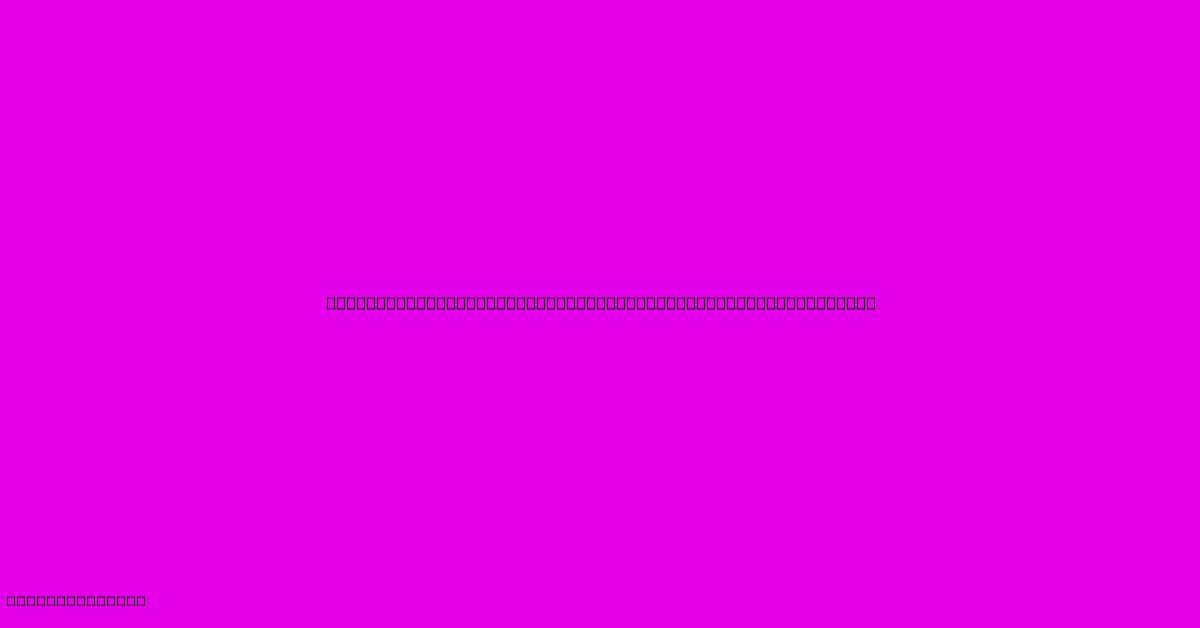
Discover more detailed and exciting information on our website. Click the link below to start your adventure: Visit Best Website mr.cleine.com. Don't miss out!
Table of Contents
The Information Phase of Technology: Data, Analysis, and the Human Element
The information phase of technology isn't about building the next groundbreaking gadget; it's about harnessing the power of the data those gadgets generate. This phase primarily requires three crucial components: data acquisition, data analysis, and human expertise. Let's delve deeper into each.
1. Data Acquisition: The Foundation of Information
This stage focuses on gathering raw data from diverse sources. Think of it as the groundwork for any technological advancement in the information age. Without robust data acquisition, the subsequent phases crumble. Key aspects include:
- Data Sources: This is incredibly broad, encompassing everything from sensor networks (IoT devices, weather stations) to databases (customer records, financial transactions), social media feeds, and scientific experiments. The more diverse and reliable the data sources, the richer the insights.
- Data Storage: Efficient and scalable storage solutions are critical. Cloud computing plays a massive role here, providing the infrastructure to handle the exponential growth of data. Big data technologies, such as Hadoop and Spark, are vital for processing and storing vast quantities of information efficiently.
- Data Integrity: Ensuring data accuracy and reliability is paramount. This involves implementing robust quality control measures throughout the acquisition process, including data cleaning, validation, and error handling. Inaccurate data leads to flawed analyses and unreliable conclusions.
2. Data Analysis: Unveiling Meaning from Raw Data
Raw data is meaningless without analysis. This stage involves transforming raw data into actionable insights. This requires sophisticated techniques and technologies:
- Descriptive Analytics: This involves summarizing and describing the data using basic statistical methods. It answers questions like "What happened?" and provides a foundational understanding of the dataset. Think of simple averages, counts, and percentages.
- Diagnostic Analytics: This digs deeper, seeking to understand why something happened. It involves identifying patterns, correlations, and anomalies within the data. Techniques include data mining and regression analysis.
- Predictive Analytics: This leverages historical data to predict future outcomes. Machine learning algorithms are commonly employed to build models that forecast trends and potential risks. Examples include fraud detection and customer churn prediction.
- Prescriptive Analytics: This goes beyond prediction to suggest optimal actions based on the analysis. This often involves optimization algorithms and decision support systems.
3. Human Expertise: The Crucial Link
Technology alone isn't enough. The information phase heavily relies on human expertise to guide the process, interpret results, and make informed decisions. This includes:
- Data Scientists: These professionals possess expertise in both statistics and computer science, enabling them to extract meaningful insights from complex datasets.
- Domain Experts: Individuals with deep understanding of the specific industry or field are crucial for contextualizing the data analysis and ensuring the results are relevant and applicable.
- Business Analysts: They bridge the gap between technical analysis and business strategy, translating data insights into actionable plans and communicating findings to stakeholders.
Challenges in the Information Phase:
The information phase is not without its challenges:
- Data Security and Privacy: Protecting sensitive data is paramount. Robust security measures are crucial to prevent breaches and ensure compliance with regulations.
- Data Bias: Data can reflect existing biases, leading to skewed results. Careful data cleaning and analysis are needed to mitigate this.
- Data Silos: Data often resides in disparate systems, hindering efficient analysis. Integrating data from different sources is a significant hurdle.
Conclusion:
The information phase of technology is a dynamic and rapidly evolving field. Its success hinges on a robust synergy between data acquisition, advanced analytical techniques, and the crucial role played by human expertise in interpreting results and translating them into tangible value. By addressing the challenges and leveraging the opportunities, we can unlock the immense potential of data to drive innovation and progress across diverse sectors.
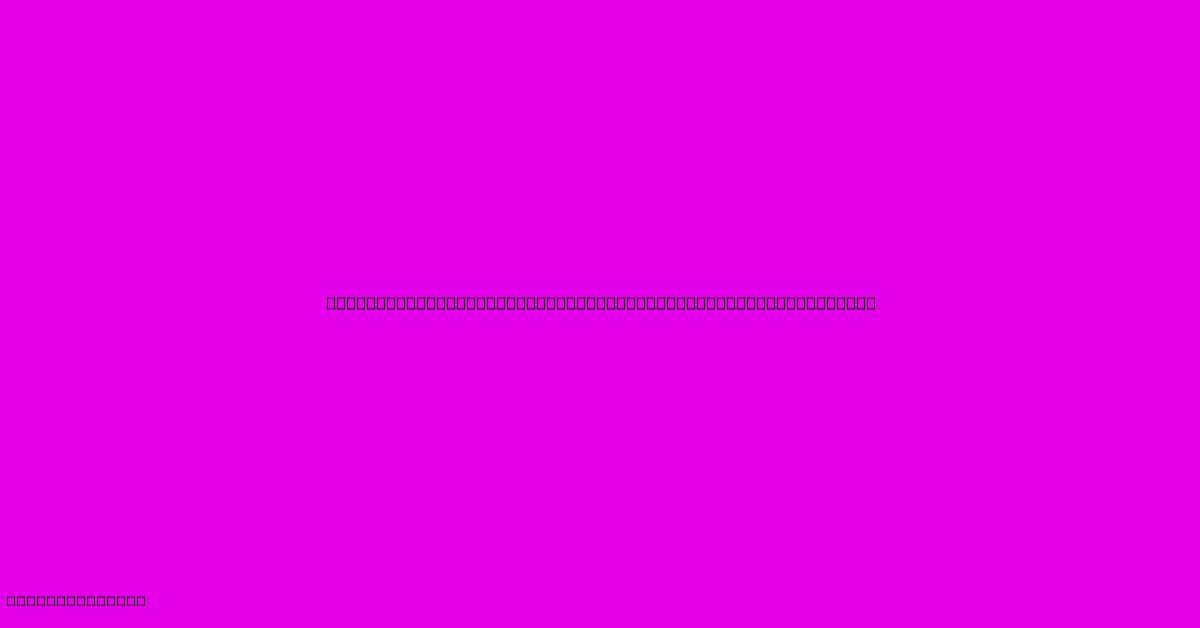
Thank you for visiting our website wich cover about The Information Phase Of Technology Primarily Requires:. We hope the information provided has been useful to you. Feel free to contact us if you have any questions or need further assistance. See you next time and dont miss to bookmark.
Featured Posts
-
Jets Rams Recap Fast Start Missed Chances
Dec 23, 2024
-
Navy Pilots Shot Down Red Sea
Dec 23, 2024
-
Game Of Thrones Fanfiction North Technology
Dec 23, 2024
-
Spadaro Analyzes Eagles Defeat
Dec 23, 2024
-
Urban Cable Technology Inc
Dec 23, 2024