Predictive Technology
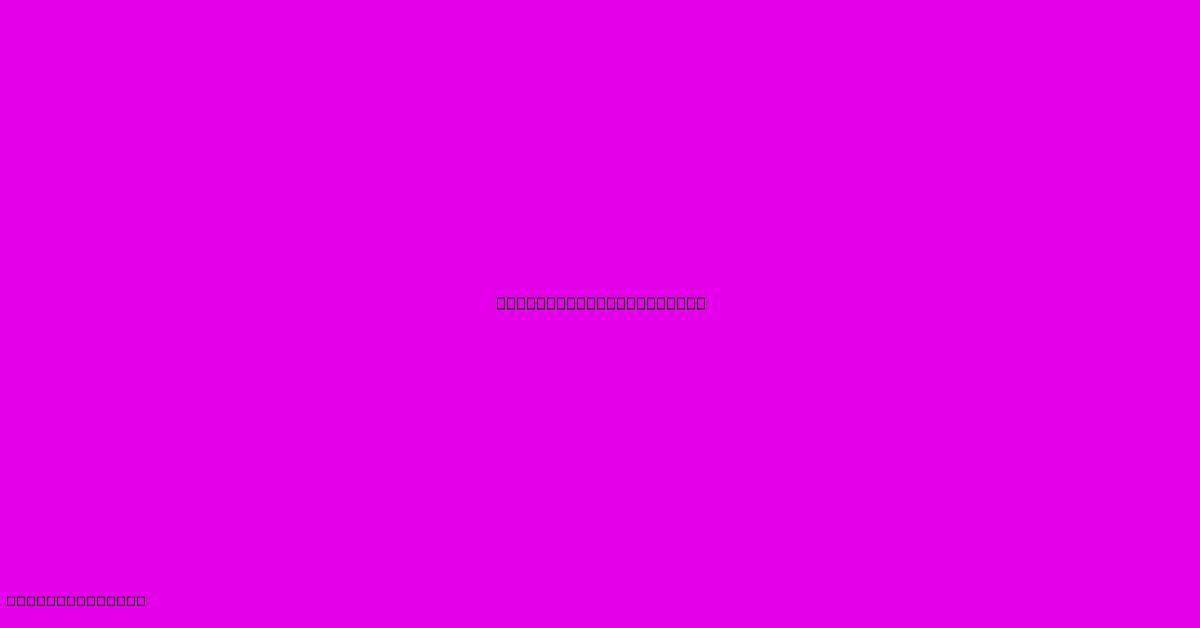
Discover more detailed and exciting information on our website. Click the link below to start your adventure: Visit Best Website mr.cleine.com. Don't miss out!
Table of Contents
Predictive Technology: Shaping Our Future, One Algorithm at a Time
Predictive technology is rapidly transforming how we live, work, and interact with the world. It leverages advanced analytics, machine learning, and artificial intelligence to analyze vast amounts of data and predict future outcomes. This isn't about fortune telling; it's about using data-driven insights to make informed decisions, improve efficiency, and mitigate risks. From personalized recommendations on Netflix to predicting equipment failures in a factory, predictive technology is quietly revolutionizing numerous industries.
What is Predictive Technology?
At its core, predictive technology involves using historical data, statistical algorithms, and machine learning techniques to identify patterns and trends. These patterns are then used to forecast future events or behaviors with a degree of probability. The accuracy of these predictions depends heavily on the quality and quantity of data used, the sophistication of the algorithms employed, and the specific context of the prediction.
Key Components of Predictive Technology:
-
Data Collection and Integration: The process begins with collecting data from diverse sources – internal databases, social media, sensor networks, and external APIs. This data needs to be cleaned, processed, and integrated to create a comprehensive and usable dataset.
-
Data Modeling and Analysis: Advanced statistical techniques and machine learning algorithms are employed to identify patterns, correlations, and anomalies within the data. This involves selecting appropriate algorithms (linear regression, logistic regression, decision trees, neural networks, etc.) based on the nature of the data and the prediction task.
-
Prediction Generation: Once the model is trained and validated, it can be used to generate predictions based on new input data. These predictions are often presented as probabilities or likelihoods, reflecting the uncertainty inherent in forecasting future events.
-
Model Monitoring and Refinement: Predictive models are not static; they need to be continuously monitored and refined. New data, changing trends, and unforeseen events can impact the accuracy of predictions. Regular updates and retraining are crucial to maintain model performance.
Applications of Predictive Technology Across Industries:
Predictive technology has far-reaching applications across a wide range of sectors:
1. Healthcare: Predicting patient readmissions, identifying high-risk individuals, optimizing treatment plans, and accelerating drug discovery.
2. Finance: Detecting fraudulent transactions, assessing credit risk, predicting market trends, and personalizing financial advice.
3. Retail: Optimizing inventory management, personalizing marketing campaigns, predicting customer churn, and improving customer service.
4. Manufacturing: Predicting equipment failures, optimizing production processes, reducing downtime, and improving supply chain efficiency.
5. Transportation: Optimizing logistics, predicting traffic patterns, enhancing safety, and improving fuel efficiency.
6. Cybersecurity: Detecting and preventing cyberattacks, identifying vulnerabilities, and improving security protocols.
Challenges and Considerations:
Despite its immense potential, predictive technology faces several challenges:
-
Data Bias: Biased data can lead to inaccurate and unfair predictions. Addressing bias is critical to ensure ethical and responsible use of predictive technology.
-
Data Privacy: Collecting and using personal data raises significant privacy concerns. Robust data security and privacy measures are essential.
-
Model Explainability: Understanding how a predictive model arrives at its predictions is crucial for building trust and ensuring accountability. "Black box" models can be difficult to interpret and may lack transparency.
-
Computational Resources: Training and deploying sophisticated predictive models can require significant computational power and expertise.
The Future of Predictive Technology:
Predictive technology is constantly evolving, driven by advancements in AI, machine learning, and big data technologies. We can expect to see even more sophisticated and accurate predictions in the future, leading to further automation, increased efficiency, and improved decision-making across various sectors. However, responsible development and deployment are crucial to harness the full potential of this technology while mitigating its potential risks. A strong focus on ethical considerations, data privacy, and model transparency will be essential as predictive technology continues to shape our future.
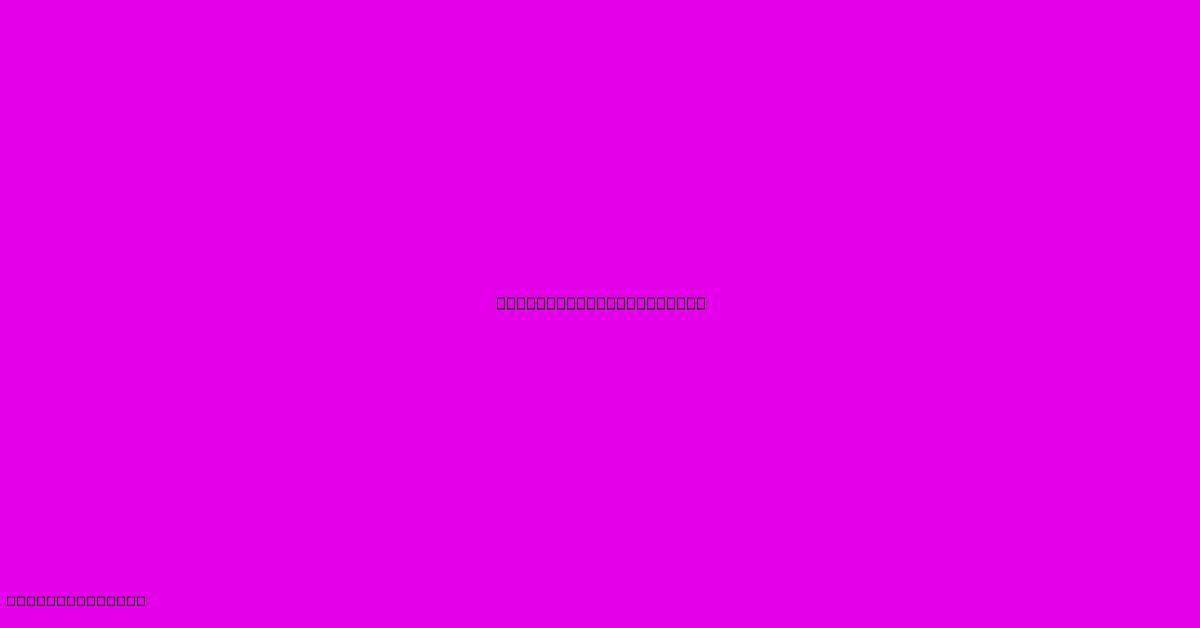
Thank you for visiting our website wich cover about Predictive Technology. We hope the information provided has been useful to you. Feel free to contact us if you have any questions or need further assistance. See you next time and dont miss to bookmark.
Featured Posts
-
Tesla Institute Of Mri Technology
Dec 21, 2024
-
Cavs X Bucks Ao Vivo Melhores Canais
Dec 21, 2024
-
Tobacco Technology
Dec 21, 2024
-
Cognizant Technology Solutions Phoenix Az
Dec 21, 2024
-
Landtag Diskutiert Foerderung Fuer Clubs
Dec 21, 2024