Oac Technology
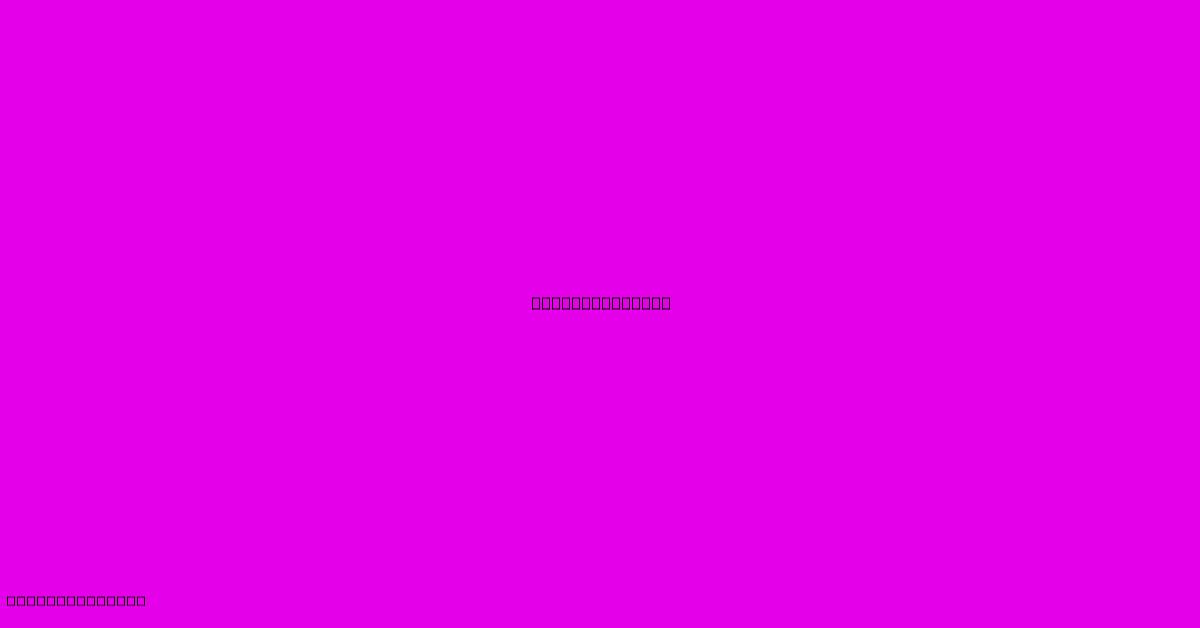
Discover more detailed and exciting information on our website. Click the link below to start your adventure: Visit Best Website mr.cleine.com. Don't miss out!
Table of Contents
Decoding OAC Technology: A Deep Dive into Object-Action Complexes
Object-Action Complexes (OACs) represent a groundbreaking advancement in the field of artificial intelligence, particularly within computer vision and robotics. This technology moves beyond simple object recognition to understand the relationship between objects and the actions performed upon them. Instead of just identifying a "knife" and an "apple," OACs comprehend the action of "cutting an apple with a knife." This nuanced understanding opens doors to a plethora of applications, far surpassing the capabilities of traditional image recognition systems.
What are Object-Action Complexes (OACs)?
At its core, OAC technology focuses on learning and representing the intricate interplay between objects and the actions they're involved in. It's not merely about detecting individual objects; it's about understanding the context of those objects within a scene and the actions they participate in. This contextual understanding is crucial for building intelligent systems capable of interacting with the real world in a meaningful way.
Think of it like this: A traditional computer vision system might identify a person, a coffee cup, and a table. An OAC system, however, would recognize the action of "the person placing the coffee cup on the table." This subtle yet powerful difference is what makes OAC technology so significant.
Key Components of OAC Technology:
Several key components contribute to the effectiveness of OAC technology:
- Object Detection: Accurately identifying and locating objects within an image or video sequence remains a fundamental building block. Advanced techniques like convolutional neural networks (CNNs) are commonly employed.
- Action Recognition: Identifying the actions being performed is equally critical. This often involves analyzing temporal information from videos, using methods such as recurrent neural networks (RNNs) or transformers.
- Object-Action Relationship Modeling: This is the core of OACs. The system needs to learn and represent the relationships between objects and the actions they participate in. This might involve creating a structured representation, such as a graph, showing the interactions between objects and actions.
- Data Representation and Learning: Effective data representation is crucial. This often involves creating datasets meticulously annotated with both object and action information, enabling machine learning models to learn the complex relationships.
Applications of OAC Technology:
The potential applications of OACs are vast and span numerous industries:
- Robotics: Enabling robots to understand and execute complex tasks involving object manipulation, like assembling products, cleaning, or performing surgery.
- Autonomous Driving: Understanding the interactions between vehicles, pedestrians, and objects on the road is essential for safe and efficient autonomous navigation. OACs can enhance the perception capabilities of self-driving cars.
- Human-Computer Interaction: OACs can improve the naturalness and intuitiveness of human-computer interaction by enabling systems to understand human actions and gestures more effectively.
- Video Surveillance: Analyzing video footage to understand events and identify suspicious activities. This involves recognizing actions like "a person breaking into a car" or "a person stealing an item."
- Healthcare: Assisting with tasks such as monitoring patient activity, analyzing medical images to identify procedures, and providing robotic assistance during surgery.
Challenges and Future Directions:
Despite its potential, OAC technology faces several challenges:
- Data Acquisition: Creating large, high-quality datasets annotated with both object and action information is time-consuming and expensive.
- Computational Complexity: Processing and analyzing the vast amount of data required for OACs can be computationally intensive.
- Robustness: OAC systems need to be robust to variations in lighting, viewpoint, and object appearance.
Future research will likely focus on:
- Developing more efficient and scalable algorithms: To handle larger datasets and more complex scenarios.
- Improving the robustness of OAC systems: Making them less sensitive to variations in the environment.
- Exploring new applications: Expanding the range of applications where OACs can be used.
In conclusion, OAC technology represents a significant leap forward in AI. Its ability to understand the relationships between objects and actions opens exciting possibilities across various domains. While challenges remain, continued research and development promise to unlock the full potential of this transformative technology.
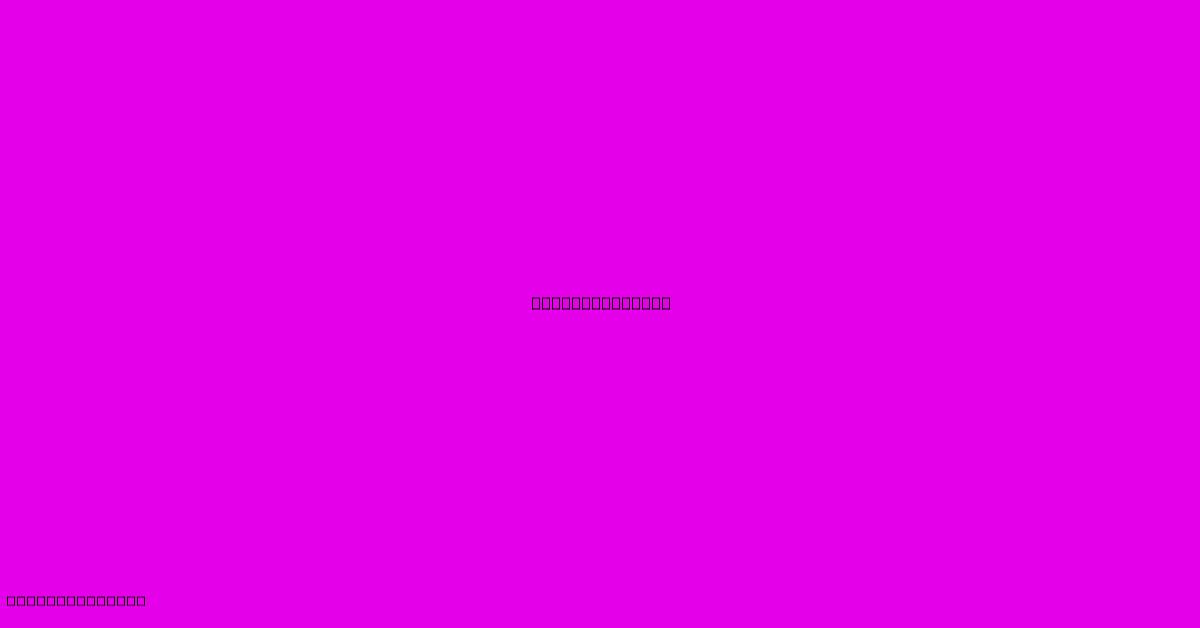
Thank you for visiting our website wich cover about Oac Technology. We hope the information provided has been useful to you. Feel free to contact us if you have any questions or need further assistance. See you next time and dont miss to bookmark.
Featured Posts
-
Premier League Liverpool Thrash Tottenham
Dec 23, 2024
-
Bournemouth Rout Manchester Uniteds Problems
Dec 23, 2024
-
Liverpool Thrash Tottenham 3 6 Epl
Dec 23, 2024
-
Wolfsburg Vs Dortmund Veja Onde Assistir
Dec 23, 2024
-
Real Madrid Sevilla Goles Y Resumen
Dec 23, 2024