How Do You Use Technology To Find The P Value
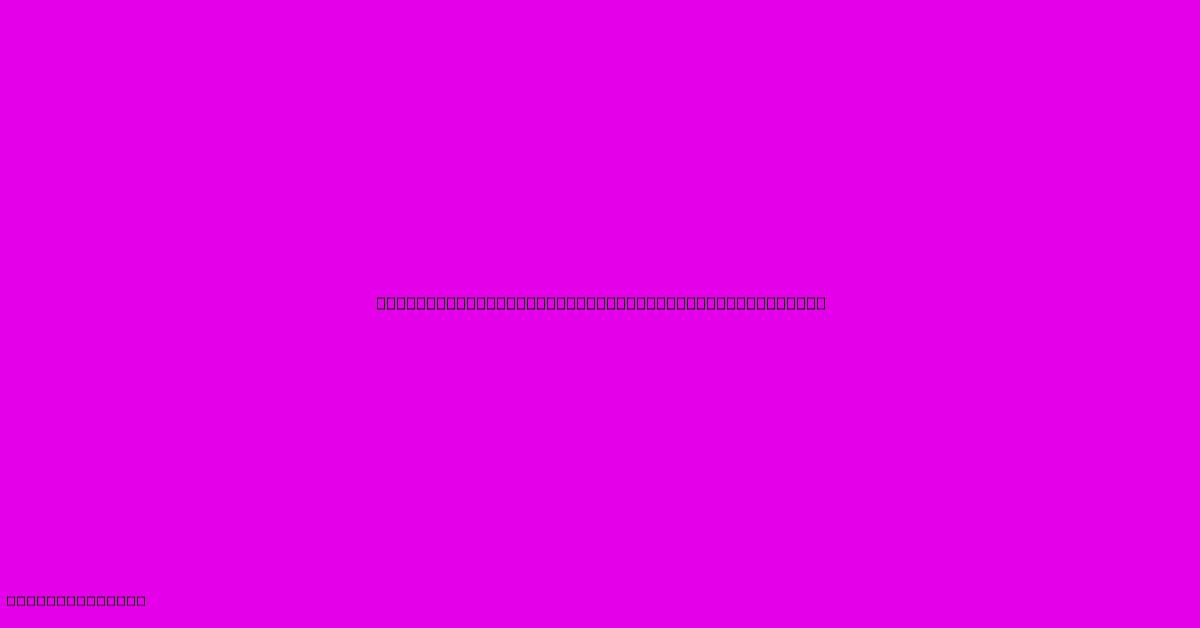
Discover more detailed and exciting information on our website. Click the link below to start your adventure: Visit Best Website mr.cleine.com. Don't miss out!
Table of Contents
How to Use Technology to Find the P-value
The p-value, a cornerstone of statistical hypothesis testing, represents the probability of observing results as extreme as, or more extreme than, the ones obtained, assuming the null hypothesis is true. Calculating p-values by hand can be incredibly tedious, especially with larger datasets. Fortunately, numerous technological tools simplify this process significantly. This article will explore how various technologies can be utilized to efficiently determine p-values.
Understanding the Context: What is a P-value?
Before delving into the technological aspects, let's briefly revisit the concept of a p-value. In essence, a small p-value (typically below a pre-determined significance level, often 0.05) suggests strong evidence against the null hypothesis, leading to its rejection. Conversely, a large p-value suggests insufficient evidence to reject the null hypothesis. The interpretation of the p-value always depends on the specific context of the statistical test being performed.
Technological Tools for P-value Calculation
Several technological tools excel at calculating p-values, catering to different statistical needs and levels of user expertise.
1. Statistical Software Packages:
-
R: A powerful and versatile open-source statistical programming language, R offers extensive libraries (like
stats
,ggplot2
) for conducting various statistical tests and obtaining p-values. Its flexibility allows for customization and complex analyses. Learning R requires investment in time, but the rewards in terms of analytical power are substantial. -
SPSS: A commercial statistical software package with a user-friendly interface, SPSS is widely used in various fields. It offers a straightforward approach to performing hypothesis tests and presenting the resulting p-values. SPSS is known for its ease of use, making it accessible even to users with limited programming experience.
-
SAS: Another commercial software, SAS is particularly popular in industries like healthcare and finance. Similar to SPSS, it provides a comprehensive suite of tools for statistical analysis and reporting, including p-value calculations. SAS is highly scalable and suitable for handling massive datasets.
-
Python with SciPy and Statsmodels: Python, combined with libraries like SciPy and Statsmodels, presents a potent alternative to dedicated statistical software. Python offers great flexibility, allowing for customized statistical analyses and integration with other data science tools. This combination is particularly attractive for researchers comfortable with programming.
2. Online Calculators:
Numerous websites offer free online calculators for various statistical tests. These calculators often require users to input the relevant data (sample size, means, standard deviations, etc.) and then provide the calculated p-value directly. While convenient, these calculators usually have limitations in terms of the variety of tests they offer and the complexity of analyses they can handle.
3. Spreadsheet Software (Excel, Google Sheets):
Spreadsheet software can handle basic statistical tests and p-value calculations. Built-in functions like T.TEST
(for t-tests) and CHISQ.TEST
(for chi-square tests) provide straightforward ways to compute p-values. However, for more complex analyses, specialized statistical software is usually preferred.
Choosing the Right Tool
The choice of technology for p-value calculation depends on various factors:
- Complexity of analysis: For simple tests, online calculators or spreadsheet software might suffice. For more complex designs or large datasets, statistical software packages are necessary.
- User expertise: SPSS offers a user-friendly interface suitable for beginners. R and Python require programming skills.
- Cost: R is open-source and free. SPSS and SAS are commercial software with licensing costs.
- Specific statistical needs: Some software packages are better suited for specific statistical methods than others.
Conclusion
Calculating p-values is integral to hypothesis testing. While manual calculations are possible, utilizing technological tools significantly improves efficiency and allows for more complex analyses. Choosing the right tool involves considering factors like analytical complexity, user skills, and budget. By leveraging the power of technology, researchers and analysts can streamline their statistical workflows and draw more robust conclusions from their data.
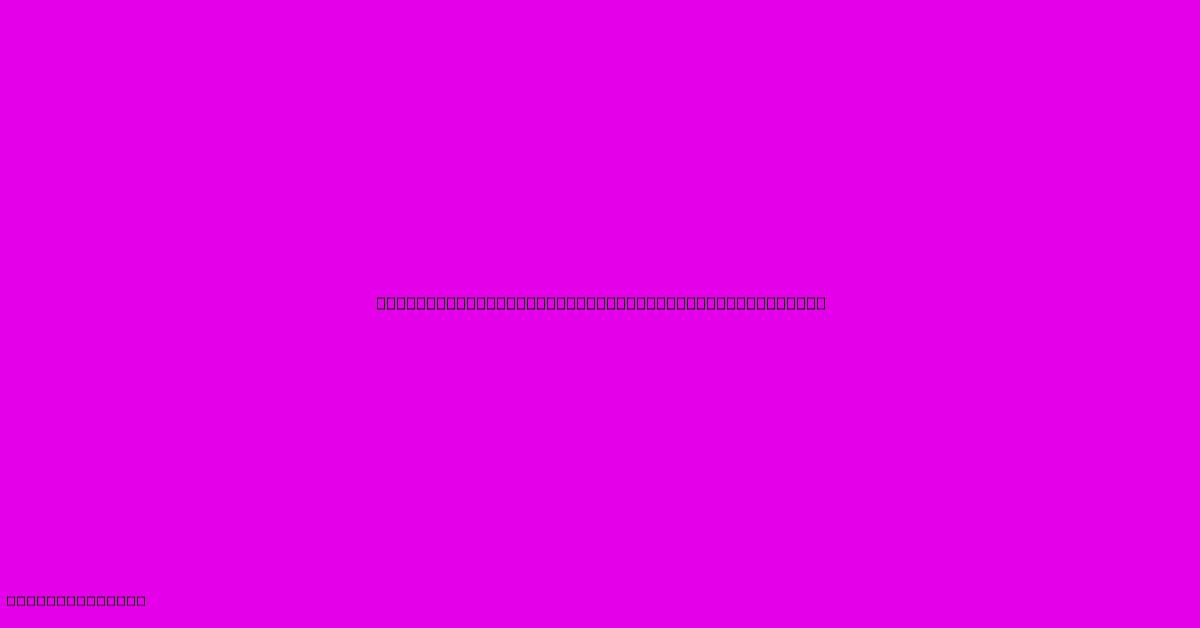
Thank you for visiting our website wich cover about How Do You Use Technology To Find The P Value. We hope the information provided has been useful to you. Feel free to contact us if you have any questions or need further assistance. See you next time and dont miss to bookmark.
Featured Posts
-
Negociacao Caixinha Gremio Encerrada
Dec 24, 2024
-
Cyclone Power Technologies Inc
Dec 24, 2024
-
Mound Technologies
Dec 24, 2024
-
Farewell Burt Crocodile Dundee Star
Dec 24, 2024
-
National Center For Preservation Technology And Training
Dec 24, 2024