Graph Adversarial Technology Experiment Log Locations
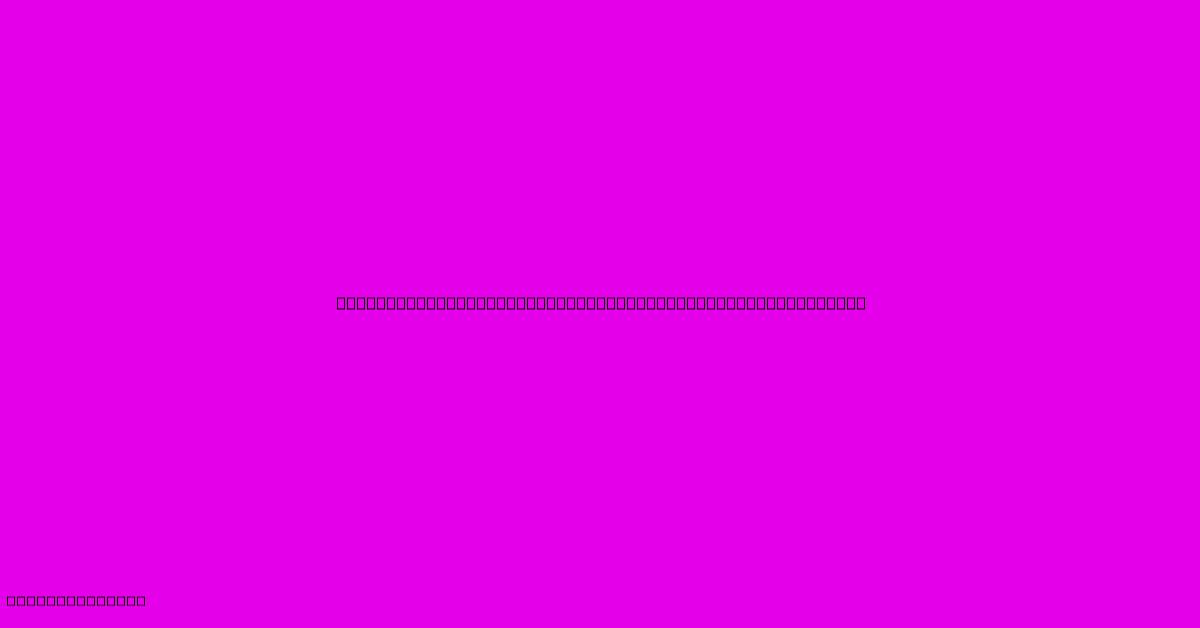
Discover more detailed and exciting information on our website. Click the link below to start your adventure: Visit Best Website mr.cleine.com. Don't miss out!
Table of Contents
Unveiling the Hidden Locations: A Deep Dive into Graph Adversarial Technology Experiment Logs
The realm of graph adversarial technology is rapidly expanding, pushing the boundaries of machine learning security and robustness. Understanding how these adversarial attacks are tested and mitigated is crucial for developing secure and reliable graph-based systems. This article delves into the often-overlooked aspect of experiment log locations – where the crucial data detailing these adversarial experiments resides.
Why Location Matters:
The location of experiment logs for graph adversarial technology holds significant weight. These logs contain invaluable information, including:
- Attack Strategies: Detailed records of the specific adversarial attacks employed, including their parameters and methodologies. This knowledge is essential for understanding attack vectors and developing robust defenses.
- Vulnerability Assessment: The logs document the vulnerabilities discovered in the targeted graph-based systems. This includes identifying weaknesses exploited by adversarial attacks.
- Defense Mechanisms: The efficacy of different defense strategies is rigorously evaluated and documented within these logs, providing crucial insights for improving system security.
- Performance Metrics: Key performance indicators (KPIs) like accuracy, precision, recall, and F1-score are meticulously recorded, allowing researchers to quantify the impact of adversarial attacks and the effectiveness of defensive measures.
- Reproducibility: Accurate log locations enable reproducibility of experiments, a cornerstone of scientific rigor and validation.
Common Locations for Experiment Logs:
The locations of these crucial logs vary depending on the research group or organization involved and the nature of the experimentation. However, some common repositories include:
- Cloud Storage Services: Platforms like AWS S3, Google Cloud Storage, and Azure Blob Storage are frequently used to store large datasets and experiment logs generated during these computationally intensive experiments. The scalability and accessibility of these services make them suitable for managing the vast amounts of data involved.
- Version Control Systems (VCS): Git repositories, often integrated with platforms like GitHub, GitLab, and Bitbucket, play a vital role in version control of code and associated data, including experiment logs. This ensures traceability and reproducibility of results.
- Local File Systems: For smaller-scale experiments, local file systems on high-performance computing (HPC) clusters or individual workstations might be employed. However, this approach is less scalable and prone to data loss.
- Specialized Research Databases: Some research groups utilize dedicated databases optimized for storing and querying experiment results, offering advanced features for data analysis and visualization.
Challenges and Considerations:
Accessing and utilizing these experiment logs present several challenges:
- Data Privacy: Logs may contain sensitive information regarding the targeted graph-based systems and the attacks themselves. Appropriate data anonymization and security measures are crucial to protect confidentiality.
- Data Format and Accessibility: The format and accessibility of the logs can be inconsistent across different research groups, hindering the ability to compare and analyze results effectively. Establishing standardized formats and metadata would greatly improve interoperability.
- Data Volume and Management: The sheer volume of data generated during these experiments can pose substantial storage and management challenges. Effective data organization and efficient query mechanisms are essential for managing these large datasets.
Future Directions:
The increasing importance of graph adversarial technology warrants a more standardized and accessible approach to experiment log management. This could involve:
- Development of standardized log formats: Facilitating easier comparison and analysis across different experiments.
- Creation of centralized repositories: Providing a central location for researchers to share and access experiment logs.
- Development of sophisticated data visualization and analysis tools: Making it easier to interpret and understand the vast quantities of data generated.
By addressing these challenges and fostering greater transparency in the sharing and management of experiment logs, the field of graph adversarial technology can progress even faster, enhancing the security and reliability of graph-based systems in various critical applications.
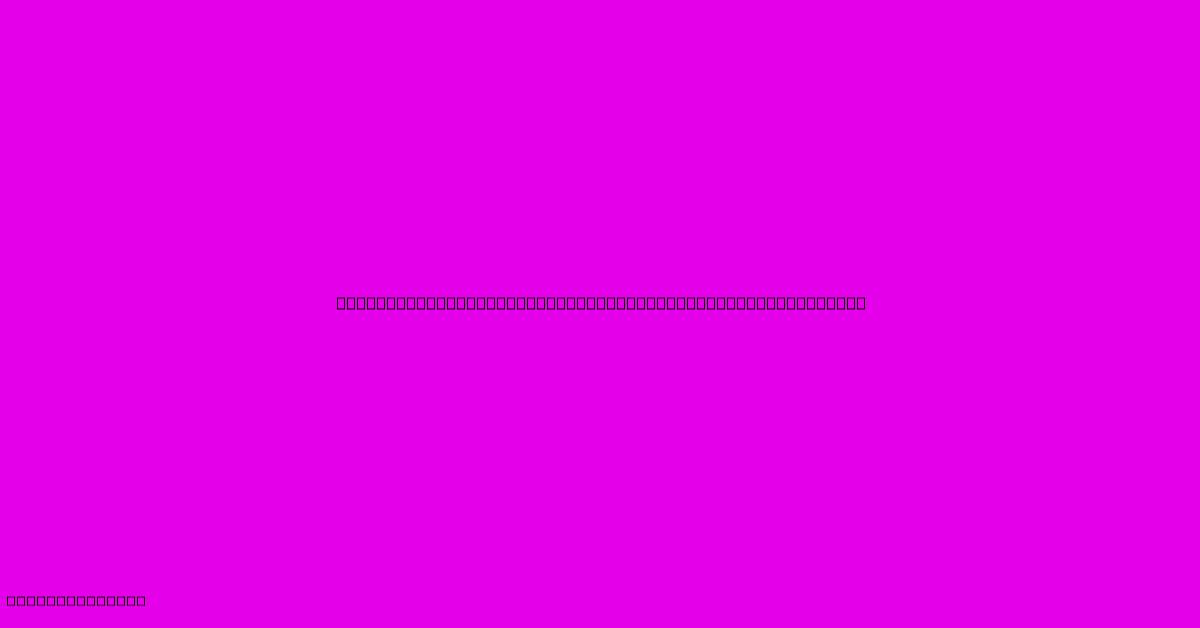
Thank you for visiting our website wich cover about Graph Adversarial Technology Experiment Log Locations. We hope the information provided has been useful to you. Feel free to contact us if you have any questions or need further assistance. See you next time and dont miss to bookmark.
Featured Posts
-
Scales Technology Academy Tempe
Dec 23, 2024
-
Concussion Hurts Misses Commanders Game
Dec 23, 2024
-
Pereiras Wolves Triumph Over Leicester
Dec 23, 2024
-
Atlanta Wins Penixs Strong Debut
Dec 23, 2024
-
Flow Technologies
Dec 23, 2024