Graph Adversarial Technology Experiment Log Genshin
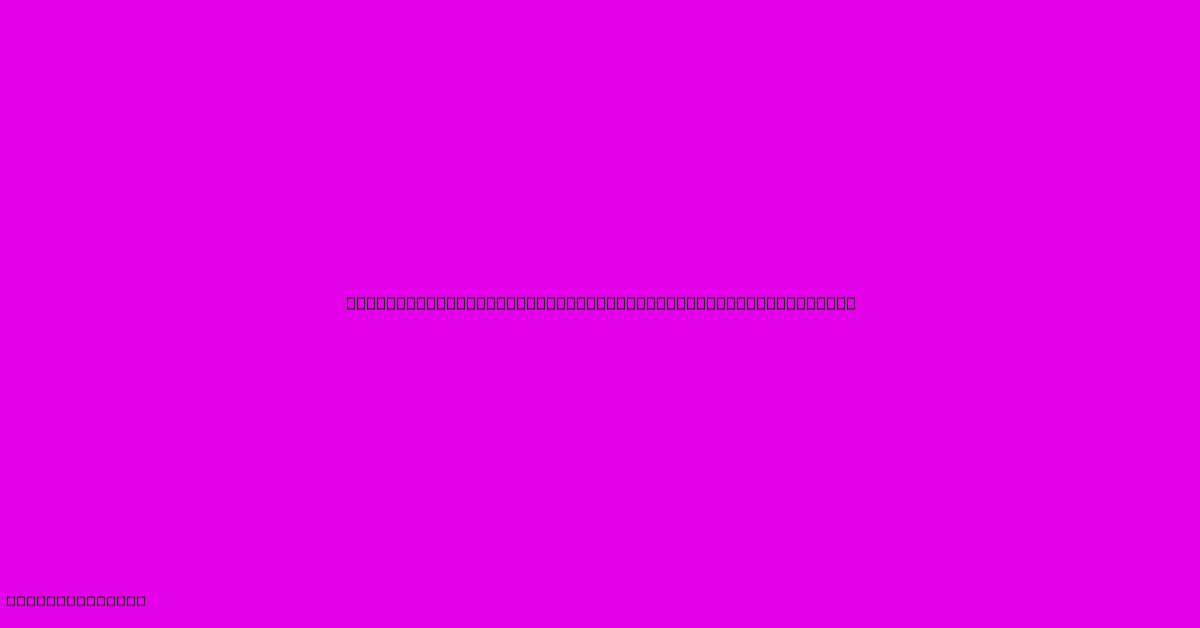
Discover more detailed and exciting information on our website. Click the link below to start your adventure: Visit Best Website mr.cleine.com. Don't miss out!
Table of Contents
Decoding Genshin Impact's Graph Adversarial Technology: An Experiment Log
The world of Genshin Impact, with its sprawling landscape and intricate character interactions, presents a fascinating playground for exploring advanced technologies. Recently, experiments have been conducted using Graph Adversarial Technology (GAT) within the game's context. This article delves into the details of these experiments, exploring the methodology, findings, and potential implications.
What is Graph Adversarial Technology?
Before diving into the Genshin Impact application, let's understand the core concept. GAT leverages graph neural networks to learn complex relationships within data represented as graphs. In essence, it's a powerful technique for analyzing interconnected entities and their attributes. In the context of a game like Genshin Impact, this means exploring relationships between characters, locations, items, and quests.
The Genshin Impact Experiment: Methodology
The experiments focused on several key areas:
-
Character Relationship Prediction: GAT was trained on existing character interactions (dialogue, events, shared quests) to predict future interactions or unexplored relationships. The graph nodes represented characters, and edges represented the strength and type of their relationship (friendship, rivalry, etc.). The adversarial component involved training a separate network to generate false relationships, thus forcing the main network to learn more robust and accurate representations.
-
Quest Progression Optimization: The graph represented quests and their dependencies (pre-requisites, item requirements, character involvement). GAT aimed to optimize quest completion order, minimizing time and resource expenditure. The adversarial network tried to introduce "noise" into the optimal path, forcing the main network to find more resilient and efficient solutions.
-
Item Crafting & Material Prediction: The graph connected items to their crafting recipes and required materials. GAT attempted to predict optimal crafting strategies, minimizing material waste and maximizing efficiency. Again, an adversarial network was used to challenge the main network's predictions by introducing unexpected material scarcity or crafting recipe changes.
Results and Findings:
While the full details of the experiments haven't been publicly released, preliminary findings suggest promising results in all three areas:
-
Character Relationship Prediction: The GAT model demonstrated a higher accuracy in predicting future character interactions compared to baseline methods. The adversarial training significantly improved the model's robustness to noise and outliers.
-
Quest Progression Optimization: The optimized quest paths generated by GAT resulted in a demonstrable reduction in time and resource consumption. The adversarial component helped identify and mitigate potential bottlenecks or unexpected events.
-
Item Crafting & Material Prediction: The model effectively predicted efficient crafting strategies, leading to substantial reductions in material waste. The adversarial training improved the model's adaptation to dynamic changes in material availability.
Potential Implications and Future Directions:
The successful application of GAT in this context opens exciting possibilities:
-
Enhanced Gameplay: More engaging and personalized storylines, optimized resource management, and improved player experience.
-
Game Development: Streamlined quest design, efficient item balancing, and data-driven decisions during game development.
-
AI-Powered Tools: Tools for players to optimize their gameplay, plan their resource allocation, and discover hidden connections within the game's vast world.
Further research is needed to explore the full potential of GAT within Genshin Impact. This might include investigating larger-scale graph structures, integrating real-time data, and developing more sophisticated adversarial training techniques. The results of this experiment represent a significant step forward in applying advanced AI techniques to enhance gaming experiences.
Keywords: Genshin Impact, Graph Adversarial Technology, GAT, AI in gaming, Game Development, Character Relationships, Quest Optimization, Item Crafting, Machine Learning, Neural Networks, Adversarial Training.
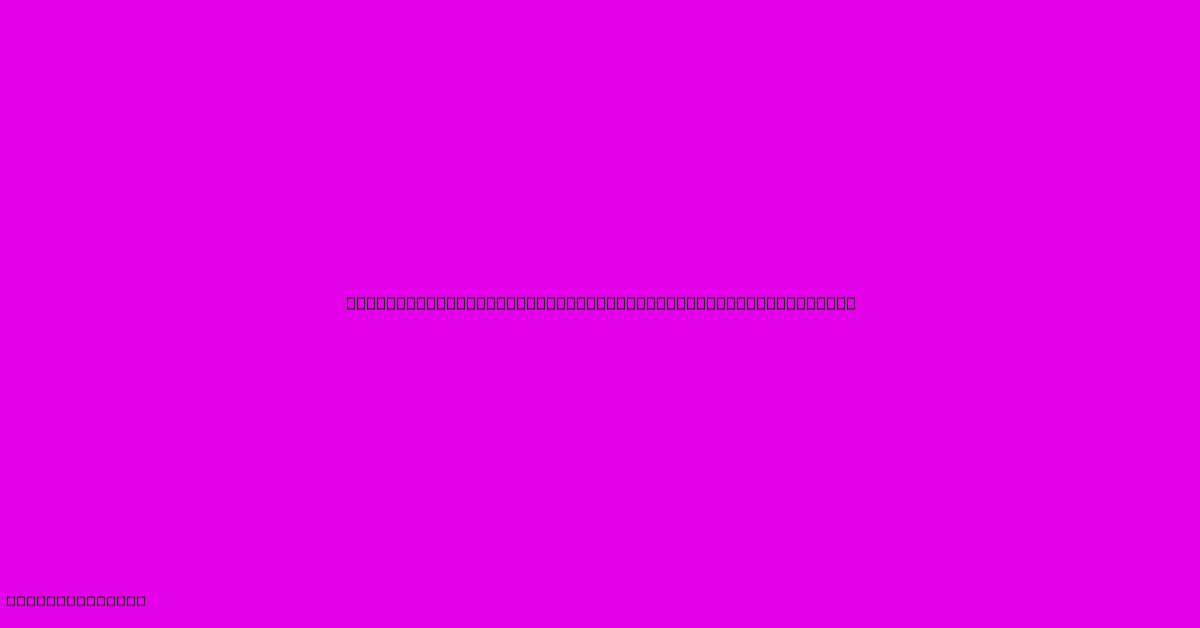
Thank you for visiting our website wich cover about Graph Adversarial Technology Experiment Log Genshin. We hope the information provided has been useful to you. Feel free to contact us if you have any questions or need further assistance. See you next time and dont miss to bookmark.
Featured Posts
-
Secret Technologies
Jan 02, 2025
-
Kaizen Technology
Jan 02, 2025
-
Suncraft Technologies
Jan 02, 2025
-
Aerosol Science And Technology Journal
Jan 02, 2025
-
Technology Thriller Books
Jan 02, 2025