Dynamic Research Technologies
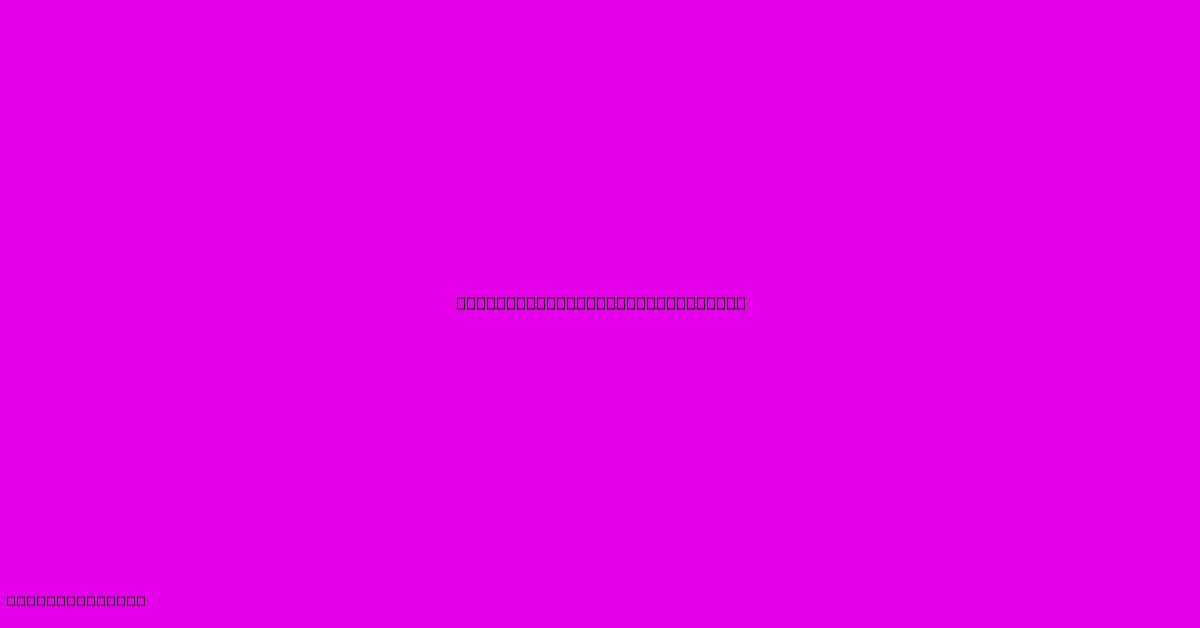
Discover more detailed and exciting information on our website. Click the link below to start your adventure: Visit Best Website mr.cleine.com. Don't miss out!
Table of Contents
Dynamic Research Technologies: Revolutionizing Data Analysis Through Dynamic Research
Dynamic Research Technologies (DRT) isn't a single, monolithic entity, but rather a broad concept encompassing numerous approaches and techniques aimed at making data analysis more agile, adaptable, and responsive to evolving needs. It's about moving beyond static, pre-planned analyses to a more fluid, iterative process that adapts as new data emerges and insights are gained. This article delves into the core principles and applications of Dynamic Research Technologies.
What is Dynamic Research?
Dynamic research contrasts with traditional research methodologies, which often follow a linear, predefined path. Traditional approaches involve formulating a hypothesis, collecting data, analyzing that data, and drawing conclusions. This process is often rigid and inflexible, unable to readily accommodate unforeseen findings or new data sources.
Dynamic research, on the other hand, is characterized by:
- Iterative Processes: Analysis is not a one-time event but a continuous cycle of data collection, analysis, refinement, and further exploration. Findings from one stage inform the next, leading to a more nuanced and comprehensive understanding.
- Adaptive Strategies: The research design itself is not fixed. It adapts and evolves based on emerging insights and the availability of new data. This allows researchers to pursue unexpected avenues of inquiry.
- Real-time Data Integration: Data is integrated and analyzed as it becomes available, rather than being collected and processed in batches. This enables quicker responses to changing conditions and trends.
- Automated Processes: Many aspects of data collection and analysis are automated, freeing up researchers to focus on interpretation and strategic decision-making.
Key Components of Dynamic Research Technologies
Several key technologies underpin dynamic research:
- Real-time Data Streaming: Technologies that allow continuous ingestion and processing of data from various sources, including sensors, social media, and transactional systems.
- Machine Learning and AI: Algorithms that can automatically identify patterns, anomalies, and trends in real-time data, providing immediate insights.
- Data Visualization and Dashboards: Interactive tools that present data in a clear and concise manner, facilitating quick understanding and decision-making.
- Cloud Computing: Scalable infrastructure that can handle the large volumes of data generated by dynamic research processes.
- Data Integration and Management: Tools and techniques for consolidating data from diverse sources and ensuring its quality and consistency.
Applications of Dynamic Research Technologies
The applications of DRT are vast and span numerous industries:
- Market Research: Understanding consumer behavior in real-time, allowing businesses to adapt their strategies quickly.
- Financial Markets: Detecting market trends and anomalies, enabling quicker and more informed investment decisions.
- Healthcare: Monitoring patient data and identifying potential problems early, leading to improved treatment outcomes.
- Supply Chain Management: Optimizing logistics and inventory management by tracking shipments and demand in real-time.
- Social Sciences: Analyzing social media data to understand public opinion and social trends.
- Environmental Monitoring: Tracking environmental changes and responding to emergencies more effectively.
Challenges and Considerations
Despite its potential benefits, dynamic research presents challenges:
- Data Volume and Velocity: Handling the sheer volume and speed of data requires robust infrastructure and efficient processing techniques.
- Data Quality: Ensuring the accuracy and reliability of data is crucial for accurate insights.
- Ethical Considerations: The use of AI and machine learning raises ethical concerns regarding bias, privacy, and transparency.
- Expertise and Skills: Implementing dynamic research requires skilled professionals who can manage and interpret complex data streams.
The Future of Dynamic Research
Dynamic research technologies are rapidly evolving, with ongoing advancements in AI, machine learning, and data processing capabilities. We can anticipate even greater automation, more sophisticated analytical techniques, and more widespread adoption across diverse fields. The future of research lies in the ability to adapt, respond, and learn continuously from ever-growing data streams. Dynamic Research Technologies is paving the way for this future.
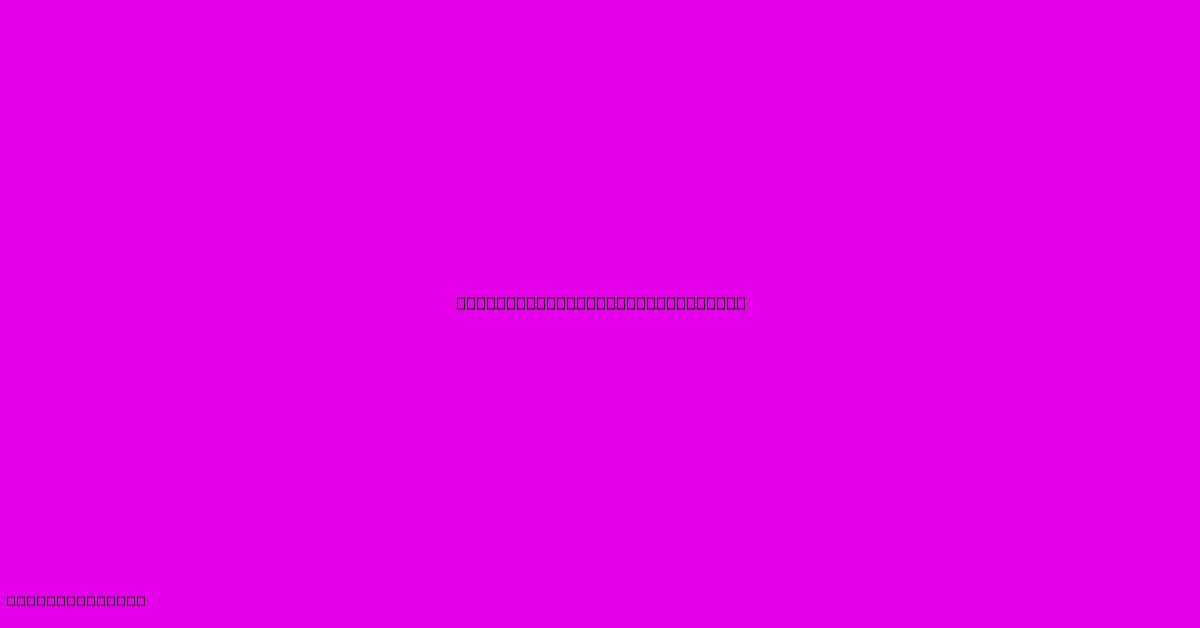
Thank you for visiting our website wich cover about Dynamic Research Technologies. We hope the information provided has been useful to you. Feel free to contact us if you have any questions or need further assistance. See you next time and dont miss to bookmark.
Featured Posts
-
Combining Two Holiday Feasts
Dec 26, 2024
-
Swift Misses Kelces Steelers Game
Dec 26, 2024
-
Usm Technology
Dec 26, 2024
-
Unity Technologies 30 3 Rd Street San Francisco Ca
Dec 26, 2024
-
Nfl Christmas Game Kelces Record
Dec 26, 2024