Dl Technology
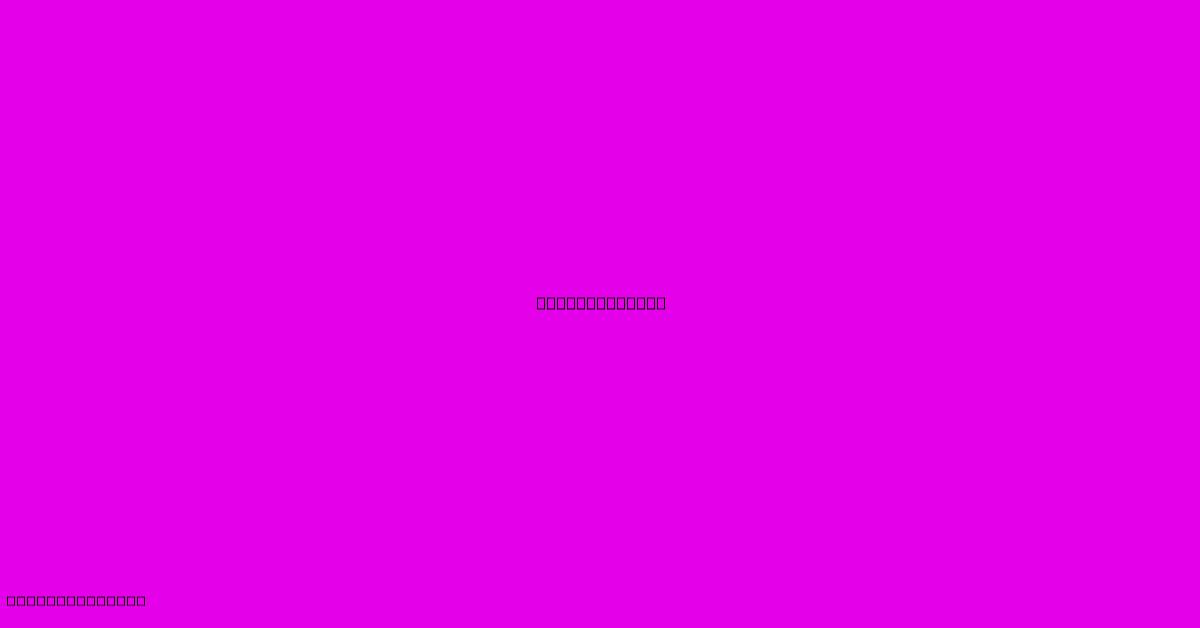
Discover more detailed and exciting information on our website. Click the link below to start your adventure: Visit Best Website mr.cleine.com. Don't miss out!
Table of Contents
Deep Dive into DL Technology: Unlocking the Power of Deep Learning
Deep learning (DL), a subfield of machine learning (ML), is rapidly transforming various sectors. This article provides a comprehensive overview of DL technology, exploring its core concepts, applications, and future prospects.
What is Deep Learning?
Deep learning leverages artificial neural networks with multiple layers (hence "deep") to analyze data and extract complex patterns. Unlike traditional ML algorithms, DL doesn't require explicit feature engineering; it automatically learns hierarchical representations from raw data. This ability to learn intricate features makes it exceptionally powerful for tackling challenging problems.
Key Components of Deep Learning:
- Artificial Neural Networks (ANNs): The foundational building blocks of DL, inspired by the structure and function of the human brain. ANNs consist of interconnected nodes (neurons) organized in layers: input, hidden, and output.
- Layers: Multiple hidden layers allow the network to learn increasingly abstract representations of the data. The depth of the network (number of layers) significantly impacts its capacity to learn complex patterns.
- Activation Functions: These functions introduce non-linearity into the network, enabling it to learn complex relationships between inputs and outputs. Examples include ReLU (Rectified Linear Unit), sigmoid, and tanh.
- Backpropagation: A crucial algorithm used to train ANNs by adjusting the network's weights and biases to minimize the error between predicted and actual outputs.
- Optimization Algorithms: These algorithms, such as gradient descent and its variants (Adam, RMSprop), guide the backpropagation process, ensuring efficient convergence towards optimal weights.
Types of Deep Learning Architectures:
Several architectures are tailored to specific tasks:
- Convolutional Neural Networks (CNNs): Excellent for image recognition, object detection, and image segmentation, leveraging convolutional layers to extract spatial features.
- Recurrent Neural Networks (RNNs): Designed for sequential data like text and time series, utilizing recurrent connections to maintain information from previous steps. Long Short-Term Memory (LSTM) and Gated Recurrent Unit (GRU) are popular RNN variants.
- Generative Adversarial Networks (GANs): Composed of two networks, a generator and a discriminator, competing against each other to generate realistic synthetic data.
- Autoencoders: Used for dimensionality reduction, feature extraction, and anomaly detection, learning compressed representations of input data.
Applications of Deep Learning:
DL's impact is pervasive:
- Image Recognition and Object Detection: Powering self-driving cars, medical image analysis, and facial recognition systems.
- Natural Language Processing (NLP): Enabling chatbots, machine translation, sentiment analysis, and text summarization.
- Speech Recognition: Used in virtual assistants, voice search, and transcription services.
- Time Series Forecasting: Predicting stock prices, weather patterns, and energy consumption.
- Recommender Systems: Personalizing recommendations for products, movies, and music.
- Drug Discovery and Development: Accelerating the identification of potential drug candidates and optimizing clinical trials.
Challenges and Future Directions:
Despite its success, DL faces challenges:
- Data Requirements: DL models typically require vast amounts of data for effective training.
- Computational Cost: Training complex DL models can be computationally expensive and time-consuming.
- Explainability and Interpretability: Understanding the decision-making process of deep learning models remains a significant challenge.
- Bias and Fairness: DL models can inherit biases present in the training data, leading to unfair or discriminatory outcomes.
Future research will focus on:
- Developing more efficient and resource-friendly DL algorithms.
- Improving the explainability and interpretability of DL models.
- Addressing issues of bias and fairness in DL applications.
- Exploring new DL architectures and applications.
Conclusion:
Deep learning is a transformative technology with immense potential to solve complex problems across various domains. While challenges remain, ongoing research and development will continue to unlock its full capabilities, shaping the future of technology and beyond. Understanding the core concepts and applications of DL is crucial for anyone seeking to navigate this rapidly evolving field.
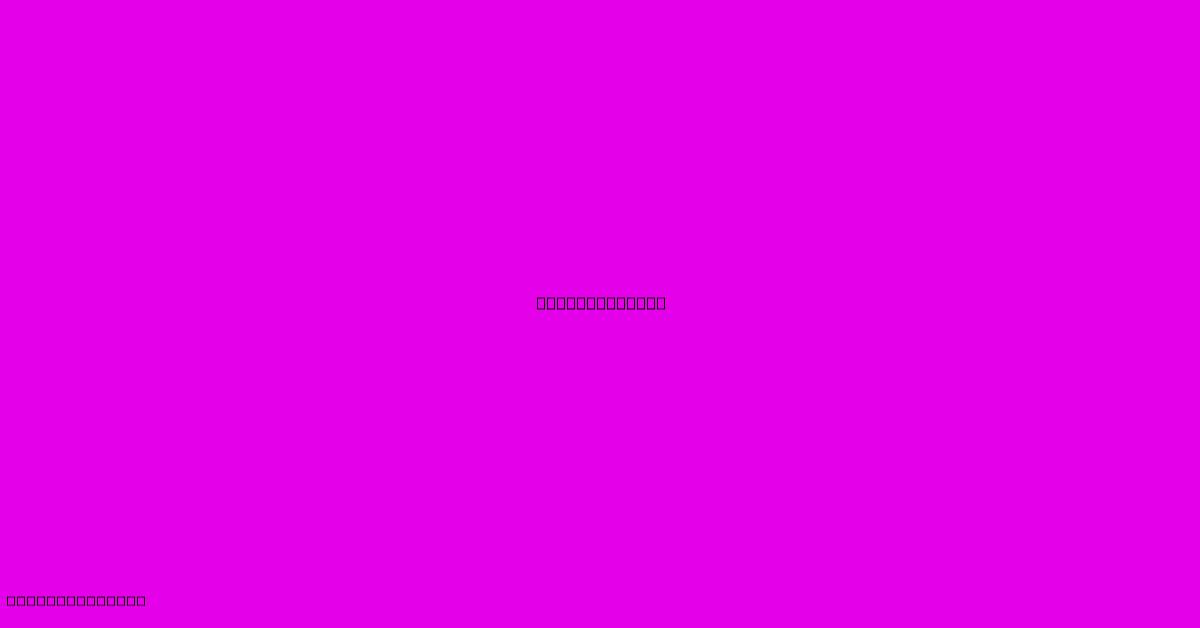
Thank you for visiting our website wich cover about Dl Technology. We hope the information provided has been useful to you. Feel free to contact us if you have any questions or need further assistance. See you next time and dont miss to bookmark.
Featured Posts
-
Muda Wa Kufungua Maduka Siku Ya Mwaka Mpya 2024
Dec 31, 2024
-
Holiday Hours New Years Day
Dec 31, 2024
-
Cranial Technologies Columbus Ohio
Dec 31, 2024
-
Oh Technologies
Dec 31, 2024
-
Arcane Technologies
Dec 31, 2024