Advanced Vision Technologies
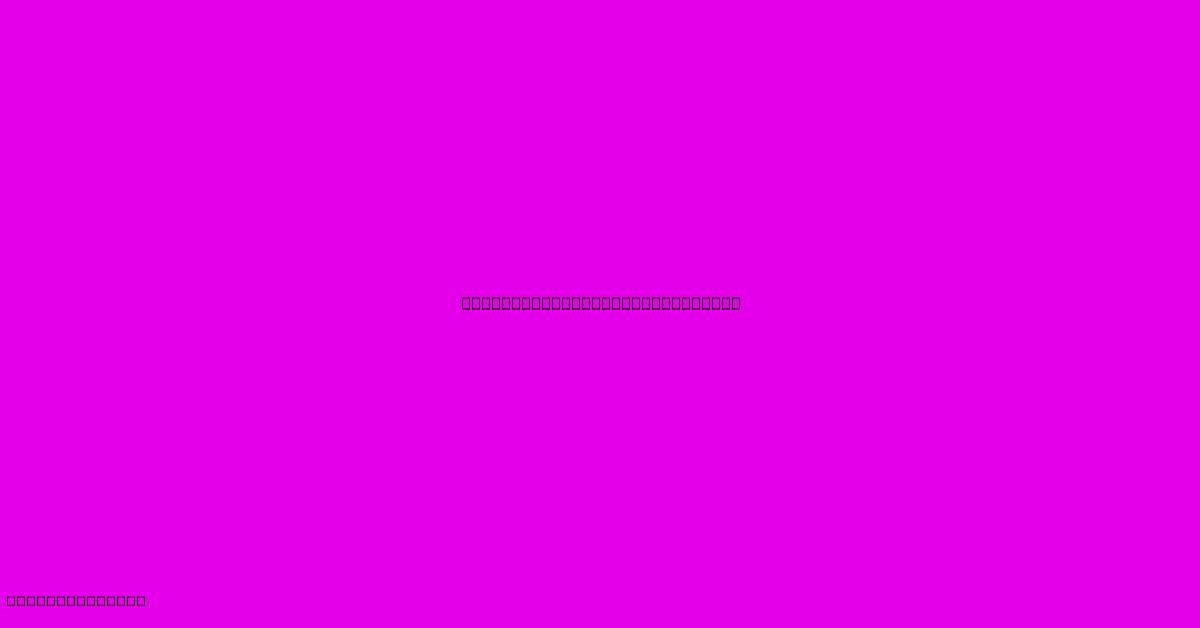
Discover more detailed and exciting information on our website. Click the link below to start your adventure: Visit Best Website mr.cleine.com. Don't miss out!
Table of Contents
Advanced Vision Technologies: Revolutionizing Perception and Interaction
Advanced vision technologies are rapidly transforming how we perceive and interact with the world. Moving beyond basic image capture, these systems leverage sophisticated algorithms and hardware to enable machines to "see" and understand their environment with unprecedented accuracy and intelligence. This article delves into the key advancements driving this revolution, exploring their applications and the future implications of this exciting field.
What are Advanced Vision Technologies?
Advanced vision technologies encompass a range of interconnected fields, including:
-
Computer Vision: This core discipline focuses on enabling computers to "see" and interpret images and videos. This involves tasks like object recognition, image segmentation, and scene understanding. Deep learning, particularly convolutional neural networks (CNNs), has been instrumental in its recent breakthroughs.
-
Image Processing: This involves manipulating and enhancing images to improve quality, extract features, and prepare data for analysis by computer vision algorithms. Techniques like noise reduction, sharpening, and color correction are crucial.
-
3D Vision: This extends beyond 2D image analysis to reconstruct three-dimensional scenes from multiple images or sensor data. This enables applications like depth estimation, 3D modeling, and object tracking in 3D space. Technologies like structured light, time-of-flight, and stereo vision are employed.
-
Embedded Vision: This focuses on integrating vision systems into smaller, more power-efficient devices. This allows for deploying vision technologies in a wider range of applications, from smart phones to IoT devices.
Key Advancements Driving the Revolution:
Several factors have propelled significant progress in advanced vision technologies:
-
Deep Learning: Deep neural networks, particularly CNNs, have drastically improved the accuracy and efficiency of object recognition, image classification, and other core computer vision tasks. This has enabled the development of more robust and reliable systems.
-
Improved Sensor Technology: Higher resolution cameras, advanced sensor modalities (e.g., thermal imaging, hyperspectral imaging), and miniaturized sensors have significantly expanded the capabilities of vision systems.
-
Increased Computational Power: The availability of powerful GPUs and specialized hardware accelerators has made it possible to process vast amounts of image data in real-time, enabling applications that were previously computationally infeasible.
-
Big Data and Cloud Computing: The ability to train deep learning models on massive datasets and leverage cloud computing resources has been essential in achieving state-of-the-art performance.
Applications Across Industries:
Advanced vision technologies are transforming numerous industries, including:
-
Automotive: Self-driving cars heavily rely on vision systems for object detection, lane keeping, and navigation.
-
Healthcare: Medical imaging analysis, robotic surgery, and disease diagnosis are being revolutionized by advanced vision techniques.
-
Manufacturing: Automated visual inspection, quality control, and robotic assembly processes are improved through advanced vision systems.
-
Security and Surveillance: Facial recognition, object tracking, and anomaly detection are used in security systems for enhanced surveillance and crime prevention.
-
Retail: Customer behavior analysis, automated checkout systems, and inventory management are enhanced with computer vision.
Challenges and Future Directions:
Despite significant progress, challenges remain:
-
Robustness and Generalization: Vision systems need to be more robust to variations in lighting, weather conditions, and viewpoints. Improving generalization capabilities to handle unseen situations is crucial.
-
Data Bias and Ethical Considerations: Bias in training data can lead to unfair or discriminatory outcomes. Addressing ethical concerns related to privacy, security, and potential misuse is critical.
-
Explainability and Transparency: Understanding how deep learning models make decisions is important for building trust and debugging errors. Developing more explainable AI is crucial.
The future of advanced vision technologies is bright. We can anticipate further advancements in:
-
Edge AI: Processing visual information directly on the device will improve real-time responsiveness and reduce reliance on cloud connectivity.
-
Multimodal Vision: Integrating vision with other sensory modalities (e.g., audio, lidar) will create more comprehensive and robust perception systems.
-
Human-Computer Interaction: Advanced vision technologies will enable more natural and intuitive interactions between humans and machines.
Advanced vision technologies are not just shaping the future of technology; they are transforming how we live, work, and interact with the world. As these technologies continue to evolve, their impact will only become more profound and far-reaching.
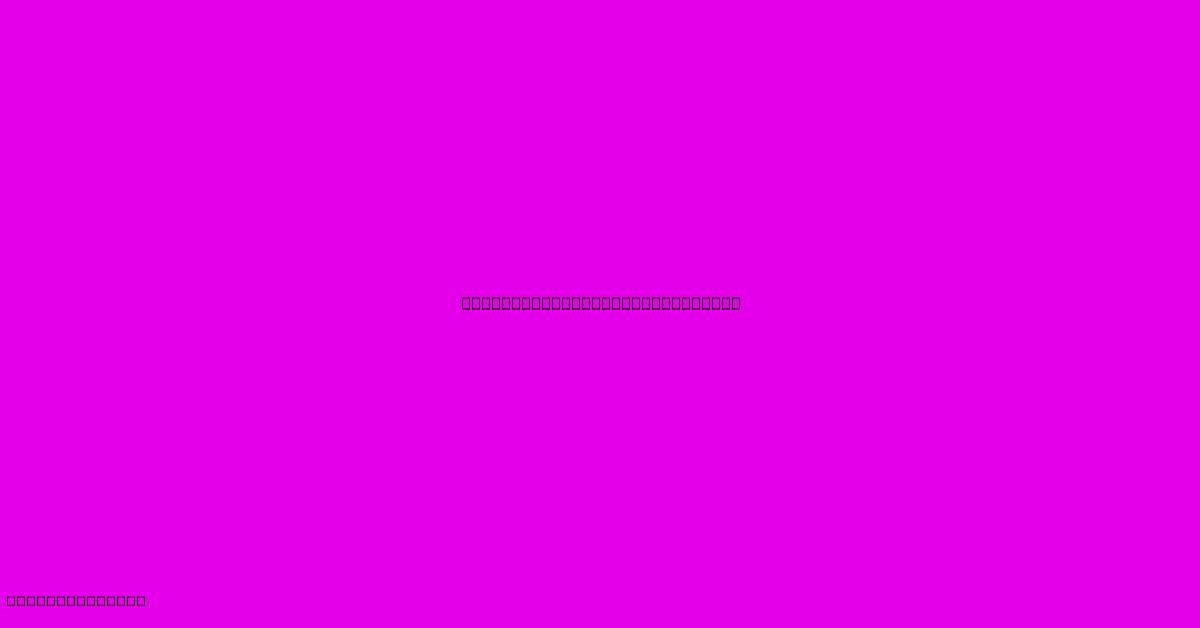
Thank you for visiting our website wich cover about Advanced Vision Technologies. We hope the information provided has been useful to you. Feel free to contact us if you have any questions or need further assistance. See you next time and dont miss to bookmark.
Featured Posts
-
Acidente Na Coreia Familiares Em Agonia
Dec 30, 2024
-
Ocean Technology Group
Dec 30, 2024
-
Assisted Reproductive Technology Essay
Dec 30, 2024
-
Nfl Week 17 Jets Vs Bills Live
Dec 30, 2024
-
Spanish Technology
Dec 30, 2024